MIT Pioneers New AI Training Approach
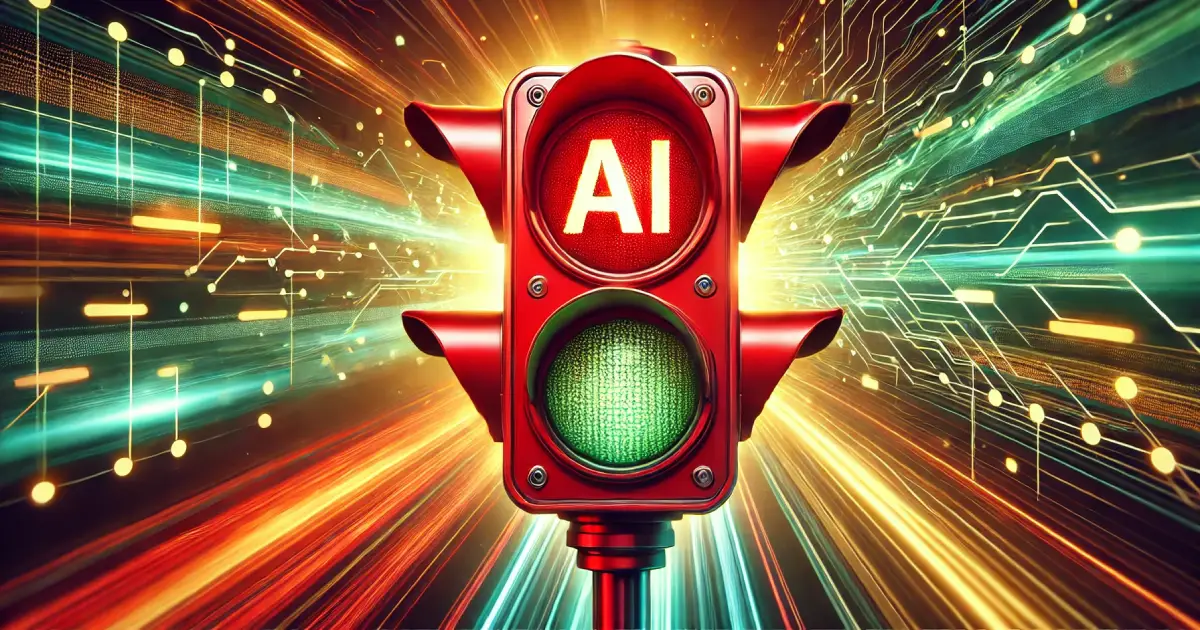
Researchers at the Massachusetts Institute of Technology (MIT) have developed an innovative algorithm to enhance the training efficiency and reliability of reinforcement learning models, particularly for complex tasks with inherent variability. This advancement holds significant potential for applications such as intelligent traffic control systems.
Challenges in Reinforcement Learning
Reinforcement learning models are designed to enable AI systems to make decisions by learning from interactions with their environment. However, these models often encounter difficulties when faced with tasks that exhibit slight variations. For instance, in traffic management, a model trained on specific intersection configurations may struggle to adapt to intersections with different speed limits, lane numbers, or traffic patterns.
MIT's Strategic Training Approach
To address this issue, MIT researchers have introduced an algorithm that strategically selects the most relevant tasks for training an AI agent. By focusing on a representative subset of tasks, the algorithm enables the AI agent to generalize its learning effectively across a broader range of scenarios. In the context of traffic signal control, this method allows the AI to perform optimally across various intersections by concentrating on those that significantly contribute to overall performance.
Efficiency and Performance Gains
The implementation of this algorithm has demonstrated remarkable improvements in training efficiency. In simulated tasks, the approach was found to be between five and fifty times more efficient than standard training methods. This increased efficiency facilitates faster learning and enhances the AI agent's performance across diverse tasks.
Implications for AI Applications
This development is particularly relevant for complex systems like urban traffic networks, where variability is a constant challenge. By enabling AI models to learn more effectively from a strategically selected set of tasks, the algorithm contributes to the creation of more reliable and adaptable AI systems.
Final Thoughts
MIT's advancement in reinforcement learning training methodologies represents a significant step toward developing AI agents capable of handling complex, variable tasks with greater reliability. This progress not only enhances the practical applicability of AI in areas such as traffic management but also sets the stage for future innovations in AI training efficiency.