The Biggest Question in AI Today
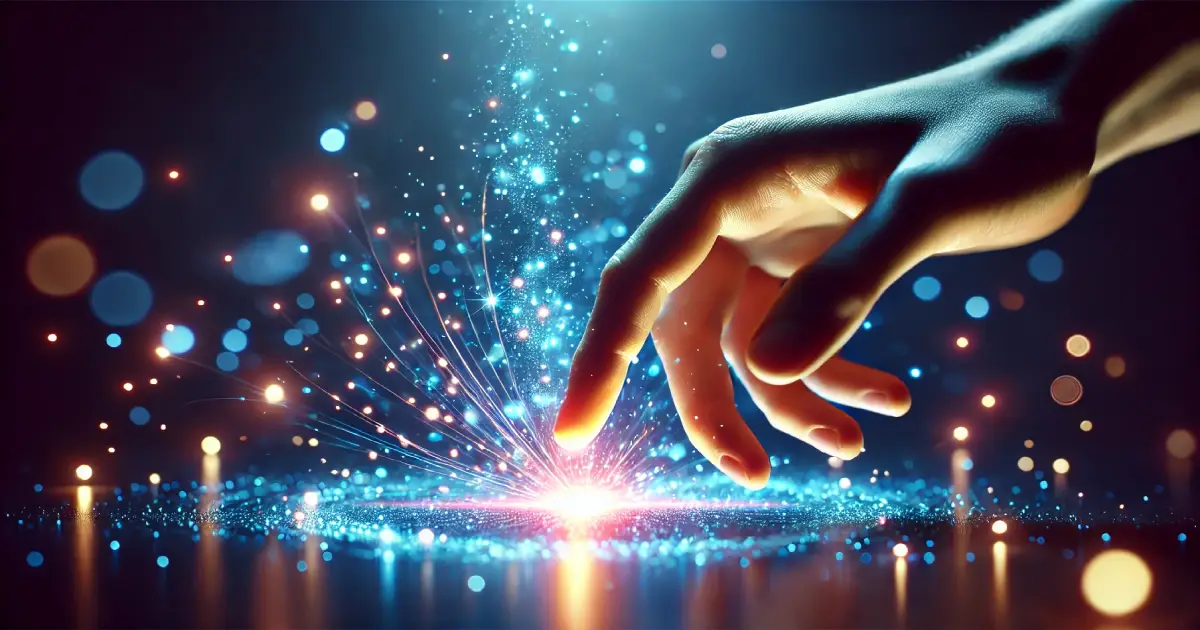
AI industry leaders are rethinking conventional strategies for model development. Historically, the focus has been on creating larger models trained on extensive datasets, operating under the assumption that more data equates to better performance. However, this method is encountering limitations, prompting a shift toward more efficient strategies.
Challenges with Data-Heavy Models
The conventional strategy of scaling AI models by increasing data volume is facing significant obstacles. The process of collecting and processing vast amounts of data is resource-intensive and may not always yield proportional improvements in model performance. Additionally, larger models often require more computational power, leading to higher costs and energy consumption.
Emergence of Smaller, Efficient Models
In response to these challenges, AI researchers and companies are exploring the development of smaller, more efficient models. These models aim to achieve comparable or superior performance with reduced data and computational requirements. Tech giants like OpenAI and Google are at the forefront of this paradigm shift, investing in research to optimize model architectures and training methodologies.
Innovative Training Techniques
Advancements in training techniques are also contributing to this evolution. Methods such as transfer learning, where a model trained on one task is adapted for another, and reinforcement learning, which focuses on decision-making processes, are gaining traction. These approaches enable models to learn more effectively from limited data, enhancing their applicability across diverse tasks.
Implications for the AI Industry
This strategic pivot has significant implications for the AI industry. By prioritizing efficiency over sheer size, companies can develop AI solutions that are more accessible and sustainable. This approach not only reduces operational costs but also minimizes the environmental impact associated with large-scale data processing.
In summary, the AI sector is undergoing a transformative shift, moving away from data-heavy models toward more efficient and adaptable systems. This evolution reflects a broader understanding that quality and efficiency can surpass quantity in advancing artificial intelligence.