The Impact of Machine Learning in Fraud Detection
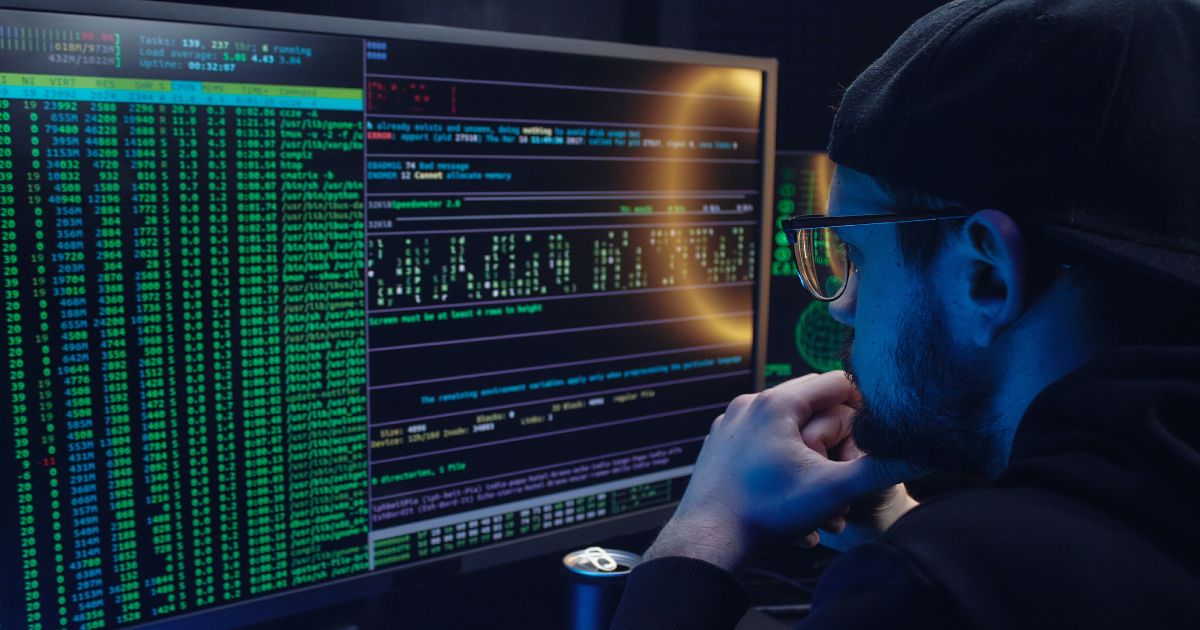
Fraud has become a significant challenge for businesses in today's digital landscape. As technology advances, so do the methods used by fraudsters to exploit vulnerabilities in various sectors.
Fortunately, the rise of machine learning (ML) has brought about a revolutionary shift in fraud detection and prevention.
By leveraging sophisticated algorithms and data analysis techniques, ML empowers organizations to stay one step ahead of fraudsters, safeguarding their assets, and protecting their customers.
The Benefits of Machine Learning in Fraud Prevention
Machine learning's ability to identify evolving fraud patterns and adapt to new threats in real-time has transformed fraud prevention strategies. By leveraging ML, businesses can proactively strengthen their security measures and respond swiftly to emerging risks.
Here are the key benefits of ML-driven fraud prevention:
1) Enhanced Accuracy
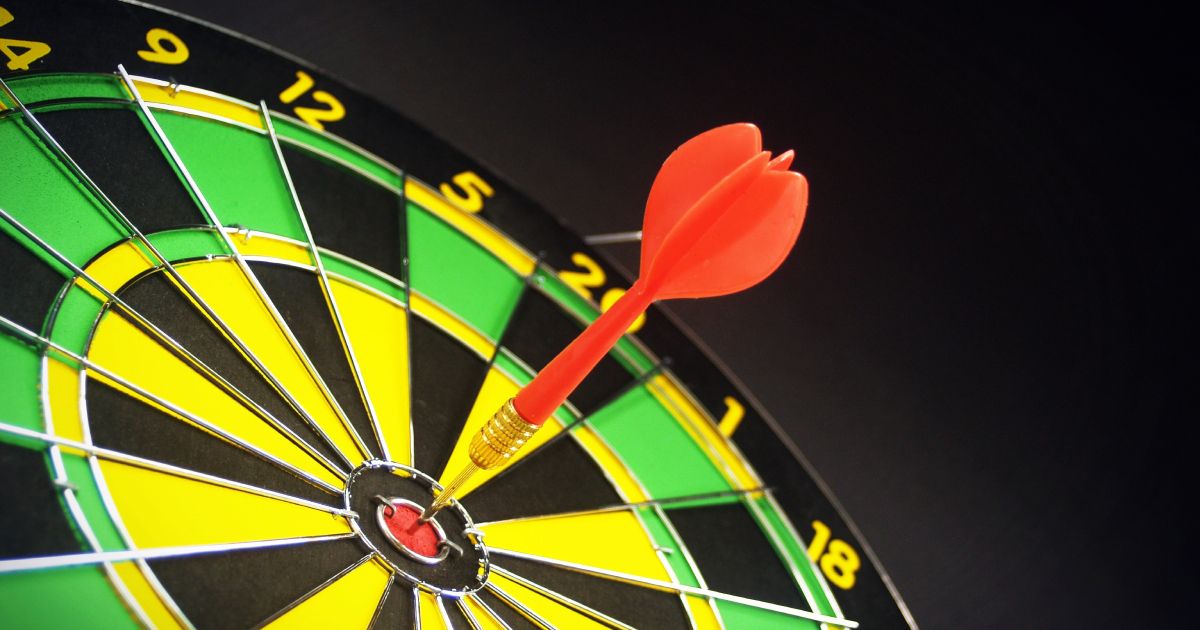
Machine learning algorithms have demonstrated exceptional accuracy in detecting fraud, making them invaluable tools for businesses seeking robust fraud prevention measures. These algorithms can analyze vast amounts of data and identify patterns that indicate fraudulent activities with high precision. By minimizing false positives, ML-powered fraud detection systems reduce the chances of overlooking legitimate transactions, ensuring that businesses can focus their resources on investigating genuine threats.
The ability of ML algorithms to discern fraudulent patterns from legitimate transactions is a game-changer for businesses. With reduced false positives, organizations can streamline their fraud detection processes, saving time and resources that would have otherwise been spent on investigating non-fraudulent activities.
ML-powered systems can swiftly pinpoint suspicious transactions, enabling businesses to respond promptly and mitigate potential risks. This level of accuracy and efficiency in fraud detection is critical for businesses operating in dynamic and high-volume environments, where quick decision-making is essential to maintain the integrity of financial transactions and protect both the organization and its customers from fraudulent activities.
2) Real-time Monitoring
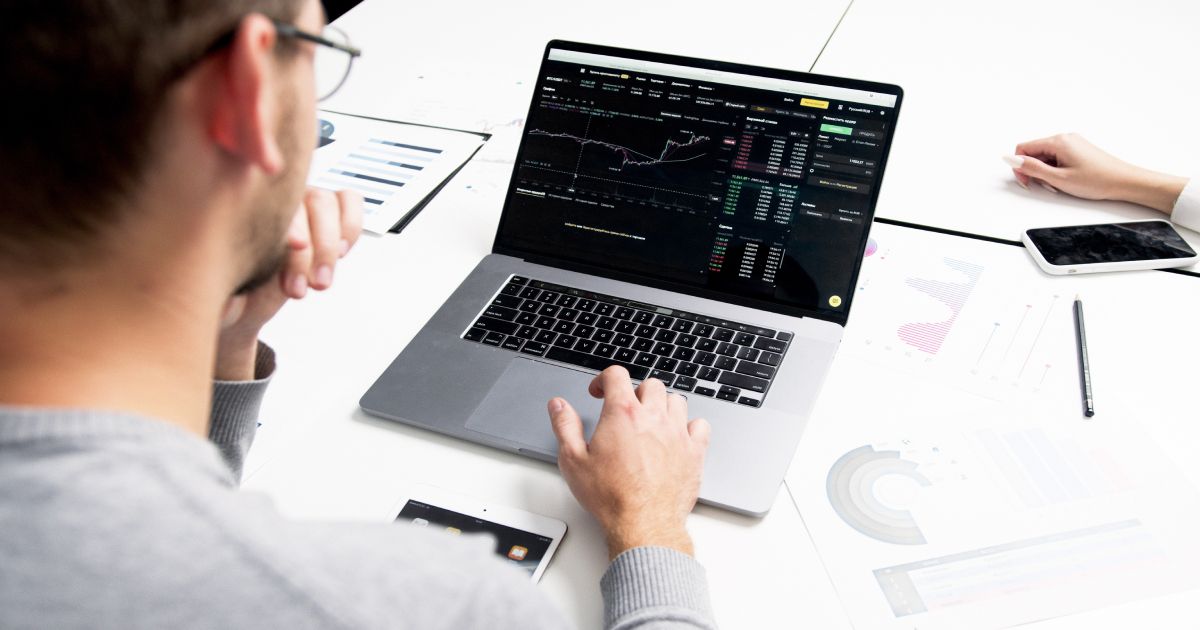
Machine learning-powered fraud prevention systems offer a significant advantage in processing vast volumes of data in real-time. By analyzing transactional data, user behavior, and other relevant information instantaneously, ML algorithms can swiftly identify patterns indicative of fraudulent activities. This real-time processing capability empowers organizations to detect and respond to fraud attempts immediately, reducing the window of opportunity for fraudsters to succeed. As a result, businesses can take timely action, preventing potential financial losses and minimizing damages to both their bottom line and reputation.
The real-time processing capacity of ML-powered fraud prevention systems is especially crucial in fast-paced and high-volume environments, such as e-commerce platforms and financial institutions, where fraudulent activities can occur at any moment. With the ability to analyze data in real-time, businesses can proactively protect their assets and customers from fraudulent transactions, ensuring a secure and seamless experience for all stakeholders.
The instant response provided by ML algorithms not only boosts the effectiveness of fraud detection but also enhances the overall efficiency of fraud prevention strategies, enabling businesses to stay one step ahead of fraudsters and maintain the trust of their customers.
3) Scalability
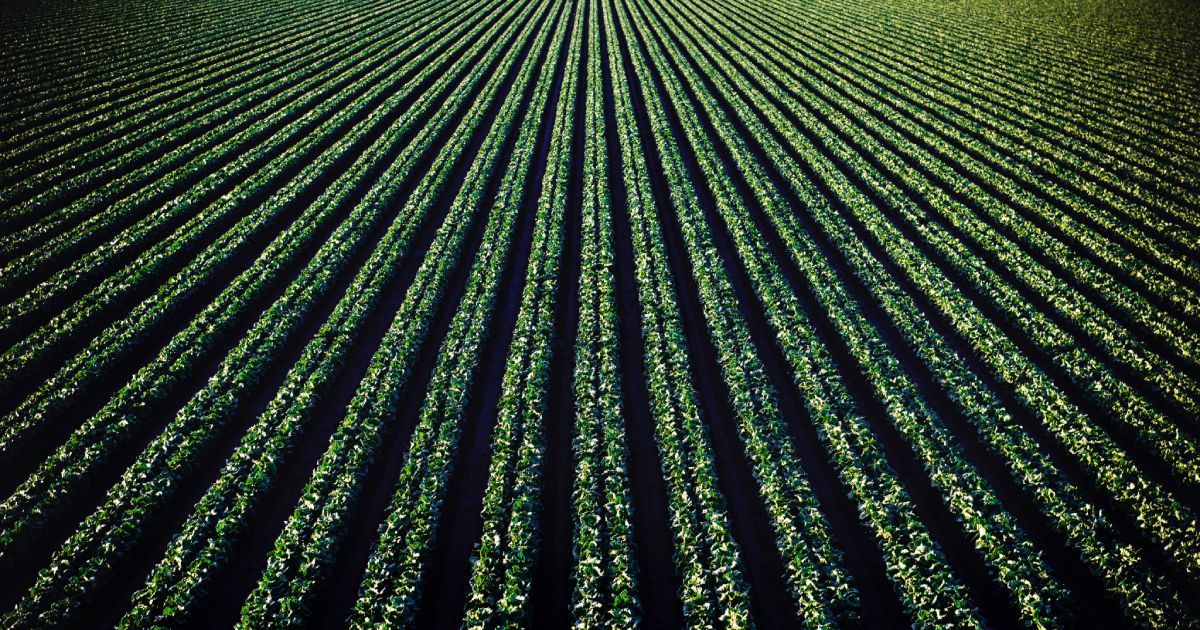
Machine learning-powered fraud detection solutions have the capability to handle vast amounts of data, making them highly adaptable and suitable for businesses of all sizes. Whether a small startup or a large enterprise, ML algorithms can efficiently process data from a few transactions to millions without compromising performance. This scalability ensures that businesses can effectively implement fraud detection measures regardless of their transaction volumes, providing consistent and reliable protection against fraudulent activities.
The ability of ML-powered fraud detection solutions to scale with the organization's needs is a significant advantage, particularly as businesses grow and face changing demands. Whether during periods of high activity or expansion, ML algorithms can effortlessly accommodate the increased data influx, ensuring that fraud detection remains robust and accurate.
This adaptability allows businesses to focus on their core operations without worrying about the limitations of their fraud detection systems, providing peace of mind and bolstering their ability to safeguard against potential financial risks and security threats.
4) Continuous Learning
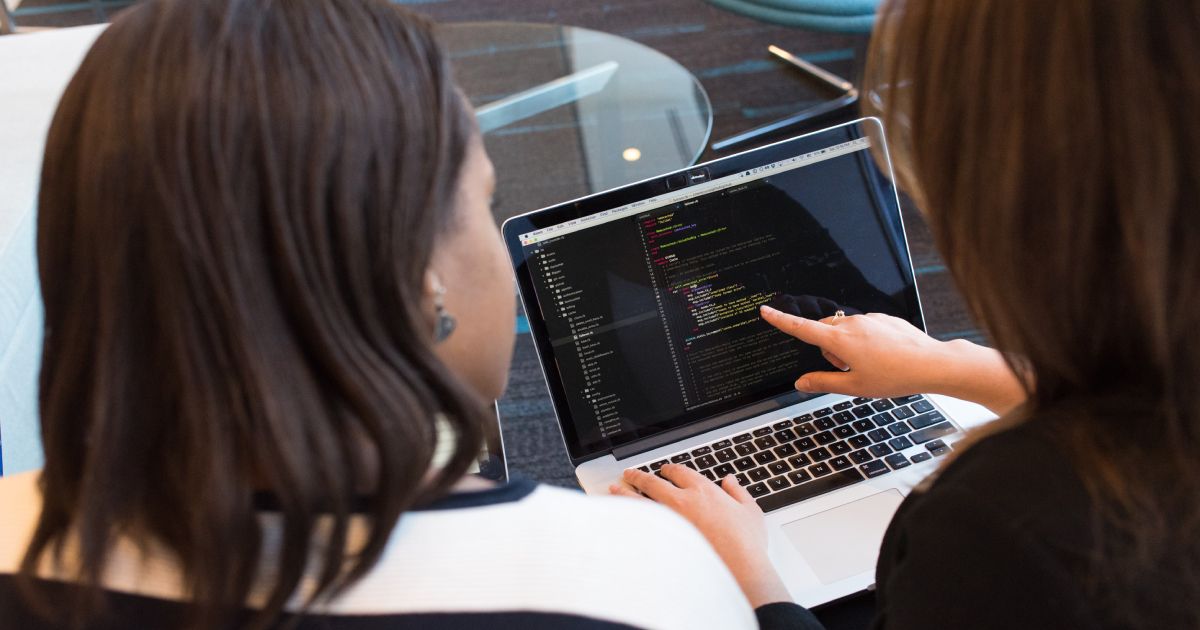
Machine learning algorithms possess a remarkable ability to continuously enhance their capabilities by learning from new data. Through ongoing analysis of transactional data, user behavior, and emerging patterns, these algorithms adapt and refine their models over time. This iterative learning process empowers the system to stay up-to-date with the latest fraud tactics and techniques, ensuring its effectiveness against ever-evolving fraudulent activities.
The adaptive nature of machine learning algorithms is a critical advantage in the fast-paced world of fraud detection. As fraudsters constantly develop new methods to bypass security measures, traditional rule-based systems may struggle to keep pace with the rapidly changing landscape.
However, ML-powered solutions can detect subtle patterns and anomalies that might indicate fraudulent behavior, even if they have never been seen before. This adaptability enables organizations to maintain a proactive stance against emerging fraud threats, fortifying their fraud prevention strategies and ensuring that their systems remain at the forefront of fraud detection technology.
Top 5 Fraud Prevention Systems
- IBM Trusteer: IBM Trusteer offers a suite of fraud prevention solutions powered by machine learning and behavioral analytics. Their advanced AI algorithms analyze user behavior in real-time to detect and prevent fraudulent activities across various digital channels, including online banking and e-commerce platforms.
- Kount: Kount's AI-driven fraud detection platform employs supervised and unsupervised machine learning models to analyze data and identify fraudulent activities. Their advanced capabilities enable merchants to differentiate between genuine customers and fraudsters, reducing false positives and optimizing fraud detection accuracy.
- DataVisor: DataVisor's unsupervised machine learning approach allows it to detect previously unknown fraud patterns, protecting businesses from sophisticated and large-scale fraud attacks. Their AI models leverage vast amounts of data to identify fraudulent activities in real-time, providing continuous protection against emerging threats.
- Feedzai: Feedzai's AI-powered fraud prevention platform analyzes vast data sets to detect anomalies and predict potential fraud before it occurs. Their robust machine learning models leverage transactional and behavioral data to improve accuracy and identify fraudulent patterns effectively.
- Forter: Forter's ML-driven fraud prevention platform utilizes real-time decision-making capabilities to detect and prevent fraud across e-commerce transactions. Their advanced AI models assess risk factors and customer behavior to offer
Final Thoughts
Machine learning is revolutionizing fraud detection and prevention, empowering businesses to proactively combat fraudulent activities.
By leveraging ML algorithms' speed, accuracy, and scalability, organizations can safeguard their assets, protect their customers, and maintain a strong defense against evolving fraud schemes.
As technology continues to advance, machine learning's role in fraud prevention will undoubtedly become even more critical, ensuring a safer and more secure digital ecosystem for businesses and consumers alike.